Analysis of Endophytic Bacterial Communities and Investigation of Core Taxa in Apple Trees
Article information
Abstract
Fire blight disease, caused by Erwinia amylovora, is a devastating affliction in apple cultivation worldwide. Chemical pesticides have exhibited limited effectiveness in controlling the disease, and biological control options for treating fruit trees are limited. Therefore, a relatively large-scale survey is necessary to develop microbial agents for apple trees. Here we collected healthy apple trees from across the country to identify common and core bacterial taxa. We analyzed the endophytic bacterial communities in leaves and twigs and discovered that the twig bacterial communities were more conserved than those in the leaves, regardless of the origin of the sample. This finding indicates that specific endophytic taxa are consistently present in healthy apple trees and may be involved in vital functions such as disease prevention and growth. Furthermore, we compared the community metabolite pathway expression rates of these endophyte communities with those of E. amylovora infected apple trees and discovered that the endophyte communities in healthy apple trees not only had similar community structures but also similar metabolite pathway expression rates. Additionally, Pseudomonas and Methylobacterium-Methylorobrum were the dominant taxa in all healthy apple trees. Our findings provide valuable insights into the potential roles of endophytes in healthy apple trees and inform the development of strategies for enhancing apple growth and resilience. Moreover, the similarity in cluster structure and pathway analysis between healthy orchards was mutually reinforcing, demonstrating the power of microbiome analysis as a tool for identifying factors that contribute to plant health.
Apples are one of the most extensively cultivated fruits globally, with a global production exceeding 86 million tons in 2019 (FAOSTAT, 2021; Liang et al., 2022). Due to their high economic and nutritional value, apples are a crucial crop for many countries. However, fire blight disease, caused by the bacterial pathogen Erwinia amylovora, has emerged as a significant threat to sustainable apple production. Fire blight can cause substantial damage to apple trees, resulting in yield losses, diminished fruit quality, and ultimately, economic losses for growers (Zhao et al., 2019). The first record of fire blight dates back to the 1780s in the United States, and since then, it has caused economic damage in many countries in Europe, North America, and more recently in Asia (Park et al., 2017). E. amylovora, which belongs to the gram-negative bacteria family Enterobacteriaceae (Breijyeh et al., 2020), causes fire blight disease in apples, pears, and other members of the Rosaceae family, thus posing a threat to the entire pome fruit industry (van der Zwet et al., 2016). The disease can spread rapidly through orchards and can infect flowers, shoots, and roots. The bacterium can survive in plant debris and soil, making it difficult to control once established in an orchard (van der Zwet et al., 2016). Moreover, the outer membrane of gram-negative bacteria can selectively retain and expel antibiotic drugs, making them more resistant to various antibiotics (McManus et al., 2002; Sundin and Wang, 2018). Copper-based bactericides have been used as a management strategy for fire blight as an alternative to antibiotics, but copper has a risk of phytotoxicity and can be harmful to animals and humans (Le et al., 2020; McManus et al., 2002). In recent years, biological agents have been suggested as a new control method for fire blight instead of copper-based bactericides or antibiotic chemicals (Massart et al., 2015; Mikiciński et al., 2020).
Alternative methods for controlling plant pathogens have gained attention in recent years. These methods rely on the use of antagonistic microorganisms that interact with the pathogen and compete for resources through hyperparasitism (Mbachu et al., 2022). Among these biocontrol agents, Bacillus subtilis BD170 (Broggini et al., 2005), Pseudomonas fluorescens EPS62e (Pujol et al., 2005), P. fluorescens A506 (Wilson and Lindow, 1993), and Pantoea agglomerans E325 (Pusey et al., 2011) have been demonstrated to suppress the fire blight pathogen. These biological agents have been isolated from the ecological niche of plants, cultivated under classical methods, and assessed for their antagonistic activity using in vitro and in vivo analyses (Köhl et al., 2019). As the occurrence of plant diseases is influenced by micro-ecological interactions, analyzing the indigenous microbiome of the host can provide insights into potential sources of natural antagonistic strains that reduce the severity of the pathogen and the genetic mechanisms underlying their beneficial functions (Köhl et al., 2019; Mbachu et al., 2022). Ultimately, this can provide a better understanding of the genetic variability of biocontrol agents and another direct mode of action used in biocontrol through ecological microbiome analysis (Köhl et al., 2019).
Microbial metabolite pathway analysis is an important tool to gain insight into the metabolic interactions, gene expression, and metabolic changes that occur between microbiota and plants in their surrounding environment (Henry et al., 2016). This analysis enables the understanding of the mechanisms by which certain microbial groups can have beneficial or harmful effects on plants. Furthermore, by analyzing changes in microbial groups during plant infection or environmental stress, the impact of these changes on plant health can be predicted. Hence, microbiota pathway analysis is critical for comprehending the intricate interactions between microorganisms and plants, leading to improvements in plant health and productivity. The present study aimed to investigate the bacterial community structure and conserved metabolic pathways in the core microbiota community of healthy apples sampled nationwide. Our findings provide new insights into the metabolic interactions and potential benefits to apples’ health and productivity.
Materials and Methods
Nationwide apple orchards sampling
A batch of healthy apple leaves and twigs was received from the Rural Development Administration (RDA) Korea. The samples had been collected from various regions, including Yeongwol, Geochang, Andong, Yeongju, Iksan, Dangjin, Yesan, Goesan, and Danyang (Supplementary Fig. 1). Supplementary Table 1 provides the geographic coordinates of each sampling location. The samples displayed no visible symptoms of fire blight infection and were confirmed to be pathogen-free by quantitative polymerase chain reaction (qPCR) analysis (Supplementary Table 2).
qPCR for fire blight diagnosis
The standard DNA obtained from E. amylovora, a virulent strain responsible for fire blight, was diluted to a concentration of 10 ng/μl. These diluted DNA samples were subjected to PCR amplification using the XENO-Erwinia amylovora Detection Kit (Xenotype, Daejeon, Korea). The PCR reaction mixture comprised 9 μl of XENO-Erwinia amylovora Detection Kit, 10 μl of XENO 2× qPCR premix, and 1 μl of the diluted DNA. The PCR amplification protocol involved an initial denaturation step at 95°C for 10 min, followed by 40 cycles of denaturation at 95°C for 30 s, annealing at 58°C for 30 s, and elongation at 72°C for 30 s. A T100TM thermal cycler (Thermo Fisher, Waltham, MA, USA) was employed for the PCR amplification. The amplified PCR product was subjected to a melting curve analysis by fully denaturing the amplicon at 95°C for 5 min, followed by slow renaturation at 80°C for 30 s, 65°C for 30 s, and 50°C for 30 s in a sequential order. The melting curve was generated by increasing the temperature from 50 to 85°C at a rate of 1°C per 5 s. HEX dye was used for the measurement of fluorescence with excitation at 535 nm and emission at 553 nm.
Microbial DNA extraction and next generation sequencing library construction
In order to construct a 16S rRNA library, microbial DNA from the endosphere was extracted. The surface of twigs and leaves was initially sterilized by immersion in 70% ethyl alcohol for 30 s, followed by a 30-s treatment with a 1% sodium hypochlorite solution. The surface was then washed twice with autoclaved distilled water and allowed to dry. The resulting dried samples were flash-frozen using liquid nitrogen and subsequently ground into a powder using a mortar and pestle. Three samples were collected from different orchards within the same region and mixed in equal amounts by the organization. The microbial DNA extraction process was carried out using 300 mg of the pooled powder and glass beads in the FastDNA SPIN Kit for Soil (MP Biomedicals, Irvine, CA, USA), which was performed using the FastPrep-24 (MP Biomedicals) set to 6.0 speed for 40 s. Microbial DNA was then bound to a DNA-binding matrix within the kit, with a protein removal step carried out in accordance with the kit manual.
The V4 region library was constructed using a MiSeq adapter-linked primer approach. The primers used were 515F (5′-TCGTCGGCAGCGTCAGATGTGTATAAGAGACAGGTGYCAGCMGCCGCGGTAA) and 805R (5′-GTCTCGTGGGCTCGGAGATGTGTATAAGAGACAGGACTACHVGGGTATCTAATCC). Peptide nucleic acid clamps were used to interrupt the amplification of apple DNA, and included pPNA (5′GGCTCAACCCTGGACAG-3′) and mPNA (5′GGCAAGTGTTCTTCGGA-3′) (Fitzpatrick et al., 2018). The PCR reaction was performed using 2× KAPA HiFi HotStart ReadyMix (Roche, Basel, Swiss) and the following components: 2.5 μl of the template (5 ng/μl), 12.5 μl of 2× KAPA HiFi HotStart ReadyMix, 1 μl of each primer (10 pmol), 2.5 μl of each clamp (750 pmol), and 3 μl of distilled water. The thermal conditions for the reaction were as follows: denaturation at 95°C for 3 min, clamp annealing at 78°C for 10 s, primer annealing at 55°C for 30 s, and elongation at 72°C for 30 s. The denaturation, two annealing steps, and elongation were repeated 30 times, followed by a final elongation step at 72°C for 5 min. The library was replicated four times.
Bacterial community analysis
The consigned Macrogen Cooperation in Seoul, Korea conducted library sequencing of the V4 region using the Illumina MiSeq platform, generating fastq format files. The primer sequences in these files were trimmed using Cutadapt (version 2.9) and reads containing any N base pairs were removed using the dada2 package (version 1.16) in R (version 4.0.3). After trimming to a minimum length with an average phred score of 30 or higher, amplicon sequence variant (ASV) clustering was performed by dada2, which uses a divisive amplicon denoising algorithm. The clustered ASVs were identified using the IDTAXA method, which has been reported to reduce over-classification using the R package DECIPHER (version 2.24.0). Statistical analysis was performed using vegan (version 2.6-4bvcc) in R, and metabolic pathway prediction was carried out using PICRUSt2 (version 2.5.0).
Results and Discussion
Confirmation of absence of E. amylovora in healthy apple orchards using qPCR
Supplementary Table 1 presents the nine orchards located across the country, which were assessed to be free of fire blight disease. Despite their diverse geographical locations and environmental conditions, as depicted in Supplementary Table 1 and Supplementary Fig. 1, these orchards exhibited dissimilar latitudes, longitude, and climate. To validate the absence of the pathogen and to establish the criteria for a healthy orchard, we employed qPCR with four replicates using total DNA extracted from leaf and twig tissues and genomic DNA from E. amylovora. The XENO-Erwinia amylovora Detection Kit was employed to compare the Ct values. A Ct value greater than 34 was interpreted as negative detection. The outcomes revealed the absence of E. amylovora in all of the leaf and twig tissues from the nine regions (Supplementary Table 2). In contrast, the qPCR assay using genomic DNA from E. amylovora as a control displayed a Ct value of 28, which was lower than the reference Ct value of 34. The results indicate that all collected samples for microbial communities are devoid of fire blight, rendering them suitable for further analyses.
Comparison of ASV richness, alpha diversity, and microbial structure between leaves and twigs endosphere
We constructed a rarefaction curve to assess the richness of ASVs for both leaf and twig endophytes across different regions. The rarefaction curve represented the total number of bacterial 16S rRNA V4 region sequences identified (Supplementary Fig. 2). The endosphere of leaves and twigs of healthy orchards were studied in order to investigate microbial diversity and community structure. The present study focused on the abundance and diversity of the endophytic communities at the family level. The families Pseudomonadaceae, Beijerinckiaceae, and Nocardiaceae were found to be the most abundant in the leaf endosphere. On the other hand, Pseudomonadaceae was the most frequently detected family in all regions of the twig endosphere. The dominance of families varied in each region, indicating the diverse distribution of endophytes (Fig. 1A). Our analysis revealed that except for Yeongju and Yeongwol, the bacterial leaf endophytes reached a plateau below 40 ASVs, while the twig endophytes generally increased up to 50–100 ASVs. In Yeongju, ASV counts were higher in leaf tissue than in twig tissue, indicating higher variability in this region. We calculated alpha diversity, using ASVs, the Shannon index, and the Simpson index, to compare the endosphere of leaves and twigs (Fig. 1B). We found no statistically significant regional similarity within the same tissue type. However, the alpha diversity of twig endophytes was consistently higher than that of leaves across all indices (Wilcoxon rank sum tests, Observed: P = 0.000, Shannon: P = 0.000, Simpson: P = 0.008).
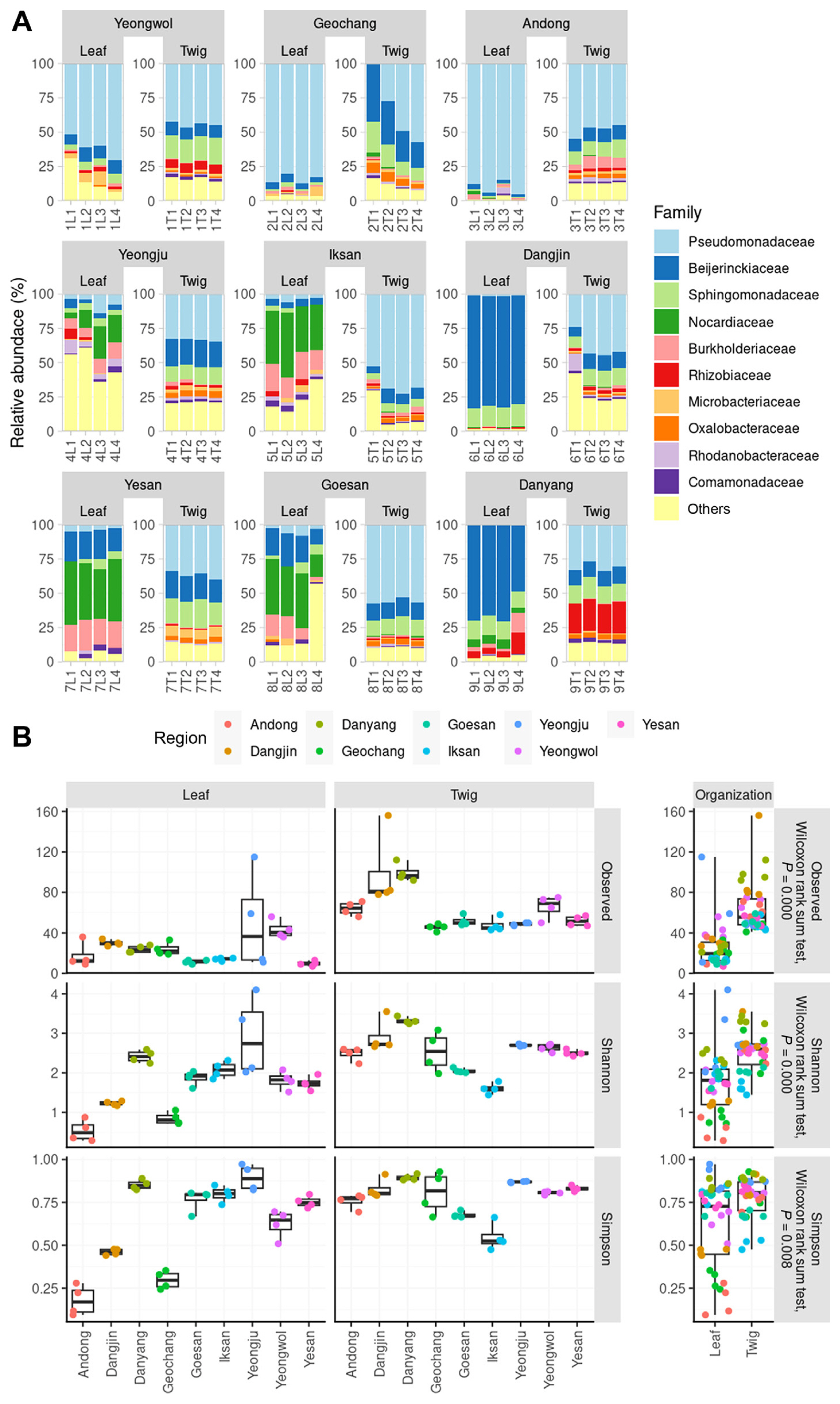
The relative abundance and alpha diversity of microbial communities in the endosphere of leaves and twigs. (A) The top 10 families in terms of relative abundance, represented as filled colors. (B) The alpha diversity of the microbial communities using a box-and-whisker plot. The left six panels show the alpha diversity values (observed amplicon sequence variants, Shannon index, and Simpson index) for each sample type. The right three panels compare the alpha diversity between the leaves and twig endosphere. Due to non-normality in the Shapiro-Wilk test, Wilcoxon rank sum tests were used for statistical analysis.
The differences in microbial community structure between regions were represented using Uniform Manifold Approximation and Projection (UMAP) and principal coordinates analysis (PCoA) based on Bray-Curtis dissimilarity (Fig. 2). The results indicated that all twig endophytes were grouped into one cluster, confirming the similarity of endophytic communities among the nine regions of the twigs. However, the leaf endophytes were divided into two or three clusters based on the regions (Fig. 2). The UMAP analysis showed that the Andong, Geochang, and Yeongwol regions were grouped together, while the remaining regions were grouped into another cluster. The PCoA analysis revealed that the Andong, Dangjin, and Yeongwol regions were grouped together, the Dangjin and Danyang regions were grouped together, and the remaining regions (Oesan, Iksan, Yeongju, and Yesan) were grouped into another cluster. These findings suggest a higher correlation between healthy orchards and twig endophyte taxa compared to leaf endophytes. Therefore, understanding the diversity and structure of endophytic communities in different regions of the orchard could help to identify and utilize beneficial microbial strains for crop improvement.

Beta diversity evaluation using Uniform Manifold Approximation and Projection (UMAP) and principal coordinates analysis (PCoA). UMAP (A) and UMAP result (B), divided into two panels for distinguishing between leaf and twig endosphere. PCoA (C) and PCoA result (D), divided into two panels for the leaf and twig endosphere. The PCoA was performed using the Bray-Curtis distance and explained statically using two-way PERMANOVA. Pairwise two-way PERMANOVA results were presented as a post-hoc test in the supplementary information.
We aimed to confirm the correlation of microbial structure among non-infected trees and identify common microbial structures that exist in nine regions by listing the taxa in the order of dominance (Supplementary Figs. 3 and 4). Our results indicate that at the family level, Pseudomonadaceae, Sphingomonadaceae, Beijerinckiaceae, Burkholderiaceae, Oxalobacteraceae, Rhodanobacteraceae, Microbacteriaceae, Rhizobiaceae, Comamonadaceae, and Solirubrobacteraceae were the top 10 taxa, on average, in twig samples (Supplementary Fig. 3). In leaf samples, Pseudomonadaceae, Beijerinckiaceae, Burkholderiaceae, Rhodanobacteraceae, Sphingomonadaceae, Nocardiaceae, Microbacteriaceae, Comamonadaceae, and Xanthomonadaceae were identified as the top 10 taxa on average, with large variation in the top taxa observed between regions (Supplementary Fig. 4). While there was a slight difference in the ranks of the average top taxa in twigs in each region, the change in ranks in leaf endophytes was relatively large, indicating a high similarity between twigs and the nine regions in the UMAP analysis. Despite the higher alpha diversity in twig tissues compared to leaves, the consistent observation of dominant bacterial taxa across samples from healthy orchards suggests that these taxa may have core functions. High diversity can facilitate various metabolic pathways within an ecological system, which can have a positive impact on plant health. Moreover, if certain clusters act dominantly despite high diversity, they may be more effective in suppressing pathogens (Xu et al., 2012).
Common taxa in the endosphere of apple leaves and twigs
A total of six common taxa were identified in the endophytic bacteria inhabiting the leaf tissue. The similarity between all regions was found to be relatively low. Four genera and two families were detected in the leaf sample, namely, Rhodococcus, Methylobacterium-Methylorobrum, Ralstonia, Pseudomonas, Sphingomonadaceae, and Comamonadaceae. The uniformly high density of any taxon in leaf tissue was not observed across all regions. However, Pseudomonas and Methylobacterium-Methylorobrum appeared to have a density of more than 50% in the same proportion as that of the twig sample. Notably, Pseudomonas was predominantly present in Andong, Geochang, and Yeongwol regions, while Methylobacterium-Methylorobrum was found in Dangjin and Danyang (Fig. 3).

Relative abundance (%) of six taxonomical groups of common bacteria found in the leaf endosphere across diverse regions. The results of the Kruskal-Wallis rank sum test indicate a significant difference (P < 0.05) among the six taxonomies. The alphabet labels represent groups that are regionally distinct, as determined by post-hoc Conover-Iman tests with a significance level of P < 0.05.
In the context of apple canker, which is caused by Neonectria ditissima, previous studies have reported differences in the structures of the endophyte communities in apple plants. Olivieri et al. (2021) observed variations in the relative abundances of specific taxa, distinguishing between diseased and healthy apples.
The endosphere of twigs was investigated to identify common and abundant bacterial genera. Pseudomonas and Methylobacterium-Methylorubrum were found to be prevalent in this environment. Additionally, the following 14 taxa, namely 1174-901-12, Sphingomonas, Burkholderia-Caballeronia-Paraurkholderia, Ralstonia, Massilia, Microbacteriaceae, Rhizobiaceae, Sphingomonadaceae, Comamonadaceae, Oxalobacteraceae, Burkholderiales, Enterobacterales, Alphaproteobacteria, and Gammaproteobacteria were also commonly observed. However, their density was considerably lower than that of Pseudomonas and Methylobacterium-Methylorubrum, as indicated in Fig. 4. Pseudomonas accounted for 25–70% of all regions, whereas Methylobacterium-Methylorubrum constituted 5–20% of the microbial community in 9 regions. These findings were consistent with the family-level taxonomic analysis. The predominance of these common taxa was observed across the endosphere of twigs, indicating that they may perform critical functions in maintaining the health of apple trees.

Relative abundance (%) of 16 common bacterial taxonomies in the twig endosphere of nine regions. The data were analyzed using the Kruskal-Wallis rank sum test, with the x-axis displaying the nine regions. Post-hoc analysis was performed using the Conover-Iman test when P < 0.05 of the Wilcoxon rank sum test. The small letters displayed above the bars indicate groups that are statistically different from one another.
Metabolic pathway enrichment of healthy twigs in apple
The present study utilized KEGG (Kyoto Encyclopedia of Genes and Genomes) enrichment analysis coupled with PICRUSt2 (Phylogenetic Investigation of Communities by Reconstruction of Unobserved States 2) to investigate the functional pathways of endophytes in non-infected apple trees for the suppression of pathogens. We aimed to identify abundant metabolite pathways that were commonly expressed in different tissues of the trees. Our results revealed dominant pathway categories, summarizing the upper metabolite pathways on an average of 20 for each tissue. The microbiomes inhabiting healthy trees showed enriched functional prediction related to fatty acid/lipid biosynthesis, such as cis-vaccenate biosynthesis, gondoate biosynthesis (anaerobic), fatty acid elongation saturated, mycolate biosynthesis, oleate biosynthesis IV (anaerobic), palmitoleate biosynthesis I (from (5Z)-dodec-5-enoate), stearate biosynthesis II (bacteria and plants), (5Z)-dodec-5-enoate biosynthesis, CDP-diacylglycerol biosynthesis I, and CDP-diacylglycerol biosynthesis II (Tables 1 and 2). We also observed that the abundance of metabolites in the twig was higher than that in the leaf, with levels ranging from 10,000 to 30,000 and below 10,000, respectively (Supplementary Figs. 5 and 6). Among the top 50 expressed pathways, we found that 46 were identical in both tissues, with the 3 highest-expressed pathways (aerobic respiration I, pyruvate fermentation to isobutanol (engineered), cis-vaccenate biosynthesis) being identical as well. However, we observed some differences in the metabolic pathways of the leaf endosphere, which had tricarboxylic acid cycle pathways regulating aerobic respiration unlike in the twig. Overall, our study provides insights into the functional pathways of endophytes in non-infected apple trees. Our findings suggest that the microbiomes inhabiting healthy trees play an essential role in regulating fatty acid/lipid biosynthesis and may contribute to the suppression of pathogens.
The present study conducted an overall pathway analysis of healthy orchards and identified the upregulation of pathways associated with fatty acid and lipid biosynthesis. In plants, fatty acids and lipids are essential components of cell membranes, playing a crucial role in regulating membrane properties and performing diverse functions, including defense mechanisms against activated cells (Lim et al., 2017). Several mechanisms have been proposed to explain how lipid metabolism in plants can contribute to antimicrobial activity and pathogen resistance. First, it is known that the expression of genes involved in fatty acid synthesis can be regulated to protect plants from pathogen attack. Increased expression of such genes leads to an accumulation of saturated fatty acids in the membrane, thereby enhancing its robustness and preventing external invasion (Lim et al., 2017). Additionally, some oxidized fatty acids, such as γ-aminobutyric acid (GABA), can negatively impact pathogen survival. GABA has been shown to possess effective toxicity against bacterial cell membranes, and an enhanced antimicrobial response in plants mediated by GABA receptors has been observed (Gramazio et al., 2020). Finally, sphingolipids, a type of lipid synthesized in plants, play a critical role in regulating plant immune responses. Specifically, ceramide and phytoceramide, two types of sphingolipids, can modulate interactions with pathogens and regulate plant responses to pathogen invasion, thus increasing plant resistance to pathogens (Zeng and Yao, 2022). The findings of this study have significant implications for improving the sustainability and health of orchard farming practices.
A comparative analysis of pathway expression rates between healthy and unhealthy apples
Evaluate the expression rate of common metabolic pathways in the healthy twig endosphere by region and compare it with tested healthy/unhealthy trees to establish the correlation between pathway expression and plant health (Fig. 5). The metabolic pathways were based on the 11 pathways identified as highly expressed in non-infected orchards from a previous study. In this analysis, the twig endophyte was compared with the previous results, as the microbial structure and pathway pattern showed similarity in the 9 regions compared to the leaves. The metabolic pathways evaluated in this study included six pathways for aromatic compound degradation (Toluene degradation III (aerobic) (via p-cresol), Catechol degradation to 2-hydroxypentanoate II, 4-methylcatechol degradation (ortho cleavage), Catechol degradation II (meta-cleavage pathway), Superpathway of salicylate degradation, Catechol degradation I (meta-cleavage pathway)), two pathways for sugar acid degradation (D-galacturonate degradation I, Superpathway of β-D-glucuronosides degradation), and one pathway each for nitrogen compound metabolism (Urea cycle), Porphyrin compound biosynthesis (superpathway of heme b biosynthesis from glycine), and Proteinogenic amino acid biosynthesis (L-isoleucine biosynthesis IV). The results indicated that, except for the MetaCyc catechol degradation I, the expression level of all metabolic pathways was statistically higher in the 9 regions of healthy orchards compared to unhealthy ones. These findings suggest that pathway analysis based on microbial structure is strongly associated with the health of plants. Furthermore, these pathways play a crucial role in maintaining the vitality and resilience of trees, and any disruptions or imbalances in these pathways could have detrimental effects on plant health (Guo et al., 2021). Therefore, further investigation is essential to comprehensively understand the mechanisms behind these pathways and develop effective strategies to promote the well-being and productivity of apple trees.

The metabolic pathway comparison of bacterial microbiota. The figure shows the pathways that are more prevalent in the healthy apple tree twig endosphere. The pink-red bars represent the results from previous studies on the twig endosphere of healthy and unhealthy apple trees, while the cyan bars represent the results from our study on nine regions of the twig endosphere. The vertical axis values indicate the gene owned by the amplicon sequence variant (ASV) index (GOO [Gene Owned by OTUs] index), which is calculated by multiplying the relative abundance by the gene counts related to the pathway of each ASV. Each GOO index is evaluated using the Kruskal-Wallis rank sum test, as depicted in the top left of the panel. The small letter above the bars depicts the Conover-Iman test.
The objective was to identify common microbial structures and pathways in healthy orchards that exhibit no detection of E. amylovora. The aim was to gain insights into factors that contribute to maintaining a healthy orchard environment. Our analysis revealed that non-pathogenic plants showed similar taxa in the twig endosphere, with a high abundance of bacterial taxa such as Pseudomonas and Methylobacterium-Methylorubrum. These findings suggest the potential significance of these bacterial groups in maintaining the health of non-pathogenic plants. Our investigation also confirmed the importance of fatty acid and lipid biosynthesis, as a common pathway shared among healthy trees. These findings provide compelling evidence that pathway analysis is a valuable indicator of plant health. In summary, our study offers valuable insights into the microbial structures and pathways associated with maintaining a healthy orchard environment, which could be useful for future studies aimed at improving orchard health and productivity.
Notes
Conflicts of Interest
No potential conflict of interest relevant to this article was reported.
Acknowledgments
This research was supported by an agenda research program by the Rural Development Administration (PJ014934).
Electronic Supplementary Material
Supplementary materials are available at The Plant Pathology Journal website (http://www.ppjonline.org/).